How Can Generative AI Solutions Be Trusted for Sensitive Applications in 2025?
Discover how generative AI can be trusted for sensitive applications in 2025 with robust security, ethics, and transparency.
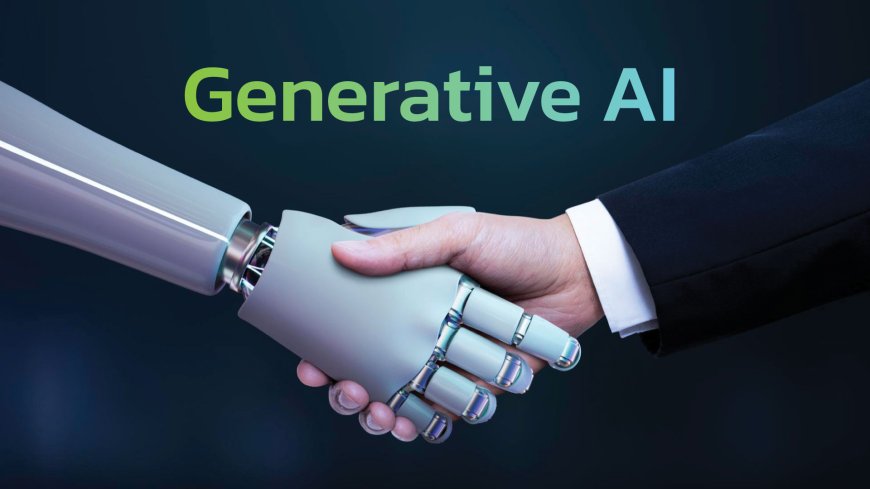
Generative AI has revolutionized industries with its ability to create text, images, and solutions at an unprecedented scale. In 2025, its applications have expanded into sensitive domains like healthcare, finance, and legal services, where accuracy and trust are paramount. However, these advancements come with unique challenges, particularly in ensuring data security, ethical compliance, and reliability.
Sensitive applications demand that generative AI not only deliver high performance but also maintain the highest standards of accountability. For example, in healthcare, an AI model generating treatment plans must adhere to strict patient confidentiality laws. Similarly, AI in financial systems must avoid biases and meet regulatory requirements. The question remains: how can these solutions be trusted in such critical environments? In this blog, we will explore the strategies, technologies, and ethical frameworks that ensure generative AI solutions can be safely and effectively deployed for sensitive applications. From transparency in AI training to advanced cybersecurity measures, the key lies in building a robust ecosystem of trust.
Defining Sensitive Applications for Generative AI
Generative AI has found its way into numerous industries, but its role in sensitive applications requires special attention. Sensitive applications refer to use cases where data privacy, ethical compliance, and precision are paramount. These include sectors like healthcare, where patient records and diagnostic insights demand utmost confidentiality, and finance, where AI-powered systems manage critical assets and transactions. Additionally, legal and governmental applications involve handling sensitive documents and public trust, leaving no room for errors or breaches.
What makes these applications particularly sensitive is the potential for far-reaching consequences in the event of a failure or ethical lapse. For instance, an error in a healthcare diagnosis can endanger lives, while a breach in financial systems can lead to significant monetary losses. Moreover, these sectors often face strict regulatory scrutiny, further emphasizing the need for generative AI systems to be secure, explainable, and reliable. Understanding these unique demands is crucial for designing AI solutions that not only perform but also inspire trust in high-stakes environments.
Building Trust Through Data Security
Data security is a cornerstone of trust in generative AI, especially for sensitive applications. Ensuring robust protections against breaches and unauthorized access is vital for safeguarding critical information. Here are key ways to enhance data security in generative AI systems:
-
Data Encryption and Secure Storage
Encrypting sensitive data ensures that even if unauthorized access occurs, the information remains unreadable. Secure storage solutions, such as cloud platforms with advanced encryption protocols, further protect data integrity and accessibility. -
Role-Based Access Control (RBAC)
Implementing RBAC restricts access to authorized personnel based on their roles and responsibilities. This minimizes the risk of insider threats and ensures sensitive data is only accessible to those who need it. -
Regular Security Audits
Conducting frequent security audits helps identify vulnerabilities in AI systems. These audits allow organizations to address potential threats proactively and maintain robust defenses against evolving cyber risks. -
Real-Time Threat Detection
Integrating AI-driven threat detection systems can identify unusual activities and potential breaches in real-time. These systems enable rapid responses, preventing small issues from escalating into significant security incidents. -
Compliance with Data Protection Regulations
Adhering to standards like GDPR, HIPAA, or CCPA ensures that generative AI solutions meet legal requirements for data privacy. Compliance builds trust with users and stakeholders by demonstrating commitment to ethical data practices. -
Data Anonymization Techniques
Anonymizing data removes identifiable information while retaining its utility for analysis. This ensures privacy is maintained without compromising the effectiveness of AI solutions. -
Continuous Security Training
Educating employees on best practices for data security helps build an organizational culture focused on protecting sensitive information. Regular training ensures that human errors, a common security risk, are minimized.
Ensuring Ethical AI Practices
Ethical AI is essential for fostering trust, particularly in sensitive applications. By addressing bias, transparency, and accountability, organizations can ensure AI systems align with societal and moral standards. Below are key practices to uphold ethical AI:
-
Mitigating Algorithmic Bias
Identifying and addressing biases in training data prevents discriminatory outcomes. Using diverse datasets and fairness-focused algorithms ensures equitable decision-making across all user groups. -
Maintaining Transparency
Providing clear insights into how AI systems make decisions fosters trust among users. Transparency tools, such as Explainable AI (XAI), allow stakeholders to understand the reasoning behind AI-generated outputs. -
Establishing Accountability Frameworks
Defining roles and responsibilities for AI development and deployment ensures accountability. This includes setting protocols for addressing errors and clarifying liability in cases of system failure. -
Implementing Ethical Guidelines
Adopting comprehensive ethical frameworks guides the development of AI systems. These guidelines ensure AI solutions align with organizational values, industry standards, and societal expectations. -
Ensuring Privacy Protection
Incorporating privacy-by-design principles ensures user data is handled securely and ethically. AI systems should minimize data collection and avoid using sensitive information without explicit consent. -
Conducting Regular Ethical Audits
Periodic reviews of AI systems help identify potential ethical risks. These audits evaluate adherence to ethical guidelines and allow for course corrections to maintain integrity. -
Promoting Stakeholder Collaboration
Engaging diverse stakeholders, including ethicists, regulators, and end-users, ensures multiple perspectives shape AI development. This collaboration helps address potential ethical concerns before deployment.
The Role of Explainable AI (XAI)
Explainable AI (XAI) is critical for building trust in generative AI systems, especially for sensitive applications. By making AI decisions transparent and understandable, XAI ensures accountability and helps stakeholders confidently adopt AI technologies. Here’s how XAI plays a vital role:
-
Enhancing Transparency
XAI enables users to understand how AI systems arrive at their decisions. This clarity fosters trust and reduces skepticism, especially in high-stakes applications like healthcare or finance. -
Facilitating Regulatory Compliance
Many industries require transparency to meet legal and regulatory standards. XAI provides the interpretability needed to demonstrate compliance with guidelines like GDPR and HIPAA. -
Improving Decision Accuracy
By exposing decision-making processes, XAI allows developers to identify and correct errors or biases in AI systems. This leads to more accurate and reliable outcomes. -
Boosting User Confidence
When users can see and understand AI reasoning, they are more likely to trust and adopt AI-driven solutions. This is particularly important in applications involving sensitive data or critical decisions. -
Supporting Ethical AI Development
XAI aligns with ethical AI practices by promoting fairness and accountability. Transparent systems ensure that biases and ethical concerns are identified and addressed promptly. -
Enabling Better Human-AI Collaboration
XAI bridges the gap between humans and AI by making systems interpretable. This collaboration allows users to validate AI suggestions and combine human judgment with AI insights effectively.
Compliance and Regulatory Frameworks
Compliance with regulatory frameworks is essential for generative AI, especially in sensitive applications. Adhering to these guidelines ensures ethical practices, data privacy, and legal integrity. Below are the key aspects of compliance and regulatory frameworks:
-
Adhering to Data Protection Laws
Frameworks like GDPR, HIPAA, and CCPA mandate strict data handling protocols. Generative AI systems must comply by securing user data, ensuring consent, and maintaining transparency in data usage. -
Implementing Privacy-by-Design Principles
Regulatory frameworks emphasize building privacy measures into AI systems from the ground up. This includes minimizing data collection and using anonymization techniques to protect sensitive information. -
Ensuring AI Explainability
Compliance often requires that AI systems be explainable and interpretable. Explainable AI (XAI) meets these demands by providing clear insights into decision-making processes, fostering trust among regulators and users. -
Meeting Industry-Specific Standards
Sectors like healthcare and finance have unique regulatory requirements. AI solutions must align with these industry-specific standards, such as FDA regulations for medical devices or PCI DSS for financial transactions. -
Establishing Accountability Mechanisms
Regulatory frameworks demand clear accountability in AI operations. Organizations must define roles, responsibilities, and protocols for addressing issues or failures in AI systems. -
Conducting Regular Audits and Assessments
Routine compliance checks and audits ensure AI systems adhere to evolving regulations. These assessments help identify gaps, address risks, and maintain long-term alignment with legal requirements. -
Promoting Ethical AI Practices
Regulatory frameworks encourage ethical AI development, including bias mitigation, fairness, and user rights. Complying with these principles ensures generative AI operates responsibly and respects societal values.
Cybersecurity Measures for Generative AI
Generative AI systems are highly innovative but also vulnerable to cybersecurity risks. Implementing robust measures ensures these systems remain secure, trustworthy, and resilient against potential threats. Here are key cybersecurity measures for generative AI:
-
Implementing Strong Data Encryption
Encrypting data at rest and in transit ensures sensitive information remains inaccessible to unauthorized entities. Advanced encryption algorithms protect AI-generated outputs and input datasets from interception or misuse. -
Adopting Multi-Factor Authentication (MFA)
MFA adds an extra layer of security to AI systems by requiring multiple verification steps. This prevents unauthorized access even if login credentials are compromised. -
Regular Security Patching and Updates
Continuously updating AI software ensures protection against emerging threats. Regular patches address vulnerabilities in algorithms, libraries, and APIs that could be exploited by attackers. -
Conducting Penetration Testing
Simulating cyberattacks through penetration testing identifies weaknesses in generative AI systems. These tests allow organizations to proactively address vulnerabilities before malicious actors exploit them. -
Integrating AI-Driven Threat Detection
AI-powered monitoring systems detect unusual activity in real-time. These tools analyze system behavior and respond quickly to potential breaches, minimizing damage and downtime. -
Restricting Access Through Role-Based Controls
Role-based access control (RBAC) limits system access to authorized users based on their responsibilities. This reduces the risk of internal threats and accidental exposure of sensitive data. -
Educating Teams on Cybersecurity Best Practices
Training employees on cybersecurity protocols ensures human errors, such as phishing or weak passwords, are minimized. A well-informed team is critical for maintaining a secure AI environment.
Continuous Learning and Adaptation in Generative AI
Generative AI thrives on its ability to learn and adapt over time. By leveraging continuous improvement, these systems stay relevant, effective, and aligned with evolving demands. Below are key aspects of continuous learning and adaptation in generative AI:
-
Leveraging Real-Time Data Updates
Generative AI systems integrate real-time data to refine their models continuously. This ensures that the outputs remain accurate, up-to-date, and reflective of current trends or contexts. -
Incorporating Feedback Loops
User feedback plays a crucial role in improving AI performance. By analyzing inputs and addressing shortcomings, AI systems can better meet user expectations and improve their outputs. -
Adapting to New Contexts
Generative AI learns to handle new scenarios by recognizing patterns and adjusting accordingly. This flexibility allows the technology to remain effective across diverse industries and applications. -
Improving Accuracy Through Reinforcement Learning
Reinforcement learning techniques enable AI to optimize its performance by learning from past actions. This iterative process helps the system adapt to complex challenges over time. -
Monitoring and Correcting Biases
Continuous learning ensures biases in generative AI models are identified and addressed promptly. Regular updates prevent discriminatory or inaccurate outputs from persisting. -
Enhancing Security and Resilience
By learning from past threats, generative AI systems improve their defenses against cybersecurity risks. This proactive approach ensures adaptability to emerging vulnerabilities. -
Scaling Capabilities with Evolving Needs
Continuous learning enables generative AI to scale effectively as demands grow. Whether handling larger datasets or accommodating more complex tasks, AI systems remain efficient and relevant.
Collaborative Efforts for Building Trust
Building trust in generative AI systems requires collaboration among developers, regulators, and users. Through shared responsibility and transparency, these efforts ensure ethical AI practices and a stronger foundation for adoption. Below are key collaborative efforts for fostering trust:
-
Engaging Multi-Stakeholder Collaboration
Collaboration between AI developers, ethicists, and industry regulators helps align AI solutions with societal values. Engaging diverse perspectives ensures AI systems address the needs and concerns of all stakeholders. -
Building Transparency with Open Communication
Regular communication with users about how AI systems work and make decisions is vital. Transparency helps demystify AI processes, enabling users to feel more confident in its outcomes. -
Establishing Ethical Guidelines and Standards
Jointly creating and adhering to ethical AI frameworks ensures systems are developed responsibly. Collaborative efforts help establish common standards for fairness, privacy, and security in AI. -
Creating User Feedback Mechanisms
Encouraging users to provide feedback on AI performance builds trust by showing that their concerns are valued. This feedback loop helps identify and resolve issues quickly, ensuring continuous improvement. -
Promoting Knowledge Sharing Across Communities
Encouraging open-source projects and knowledge sharing allows AI solutions to be developed and refined collectively. This collaborative approach enhances the reliability and effectiveness of generative AI systems. -
Developing Cross-Industry Partnerships
Collaboration between different industries, such as healthcare, finance, and technology, ensures that AI solutions are tailored to specific needs. Cross-industry partnerships foster trust by addressing sector-specific challenges and ensuring AI’s adaptability. -
Fostering Regulatory and Industry Partnerships
Collaborating with regulatory bodies ensures that AI systems meet legal requirements. These partnerships help keep AI technologies in check, balancing innovation with accountability.
Future Trends in Generative AI for Sensitive Applications
As generative AI continues to evolve, it is poised to play an increasingly vital role in sensitive applications. The future promises greater efficiency, security, and ethical considerations. Below are the key future trends in generative AI for sensitive applications:
-
Enhanced Data Privacy and Security
Future generative AI systems will integrate more robust privacy measures, including advanced encryption and secure multi-party computation. This ensures sensitive data remains protected while still allowing AI to function effectively. -
AI-Driven Regulatory Compliance
AI will increasingly assist organizations in staying compliant with evolving data protection laws. Future systems will be designed to automatically adapt to new regulations, ensuring continuous compliance without manual intervention. -
Bias Mitigation and Fairness Algorithms
With greater focus on ethical AI, generative AI systems will evolve to identify and eliminate biases. The development of advanced fairness algorithms will ensure that AI systems operate impartially, particularly in sensitive sectors like healthcare and finance. -
Integration of Explainable AI (XAI)
The trend toward explainable AI will continue to grow, with more systems offering clear and interpretable decision-making processes. This will be critical in industries where accountability and transparency are paramount, such as law and medicine. -
Collaboration Between AI and Human Experts
Future generative AI systems will work more closely with human experts, combining AI’s capabilities with human judgment. This collaboration will ensure more accurate, ethical, and responsible decision-making in sensitive fields. -
Real-Time Monitoring and Adaptation
AI systems will evolve to offer real-time monitoring and adaptive learning, enabling them to detect anomalies or ethical concerns instantly. This will improve the responsiveness of generative AI in high-stakes applications, such as cybersecurity or financial auditing. -
AI-Driven Personalization with Ethical Boundaries
Generative AI will continue to offer personalized solutions, but with strict ethical guidelines to protect user privacy. Future AI systems will be designed to respect personal boundaries while delivering highly tailored and valuable insights.
Conclusion
The future of generative AI in sensitive applications hinges on trust, which is built on a foundation of transparency, ethics, and accountability. As AI becomes more integrated into critical systems, stakeholders must ensure that these technologies are rigorously tested and regulated. Proper documentation, audits, and explainable AI models can bridge the gap between innovation and responsibility.
Moreover, collaboration between policymakers, technologists, and industry leaders is essential to create guidelines that safeguard data while encouraging innovation. Generative AI holds immense potential, but its trustworthiness in sensitive applications requires ongoing vigilance. Organizations must invest in robust cybersecurity measures, continuous monitoring, and adherence to ethical principles to mitigate risks effectively. By addressing these concerns, generative AI can transform sensitive sectors while maintaining public confidence. The key is not just technological advancement but also fostering a culture of accountability and collaboration to navigate the challenges of 2025 and beyond.
What's Your Reaction?


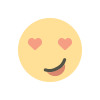
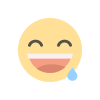
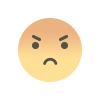
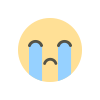
